Many non-antibiotic drugs are known to disrupt the human microbiome but their mechanisms of toxicity are unclear. Writing in Science, Guillen et al. use high-throughput genetic screens and network analysis to investigate how nearly 100 non-antibiotics inhibit bacterial growth. They uncover toxicity mechanisms that differ from conventional antibiotics, therefore implicating novel drug targets and leads for antibiotic development. The analysis also highlights how non-antibiotics could promote resistance to antibiotics.
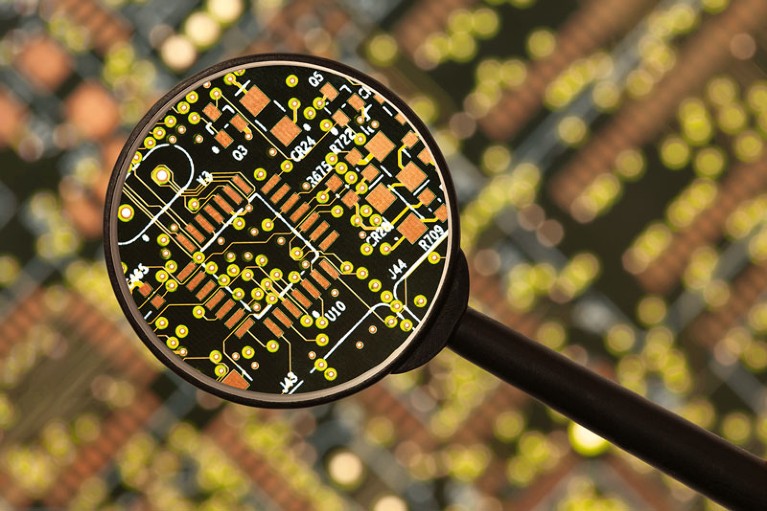
Credit: DustyPixel/E+
The study was inspired by a previous publication that revealed that a quarter of non-antibiotic drugs inhibit the growth of bacterial species from the gut microbiome. “We became fascinated to understand why these drugs are toxic to bacteria and how they kill bacterial cells”, explains senior author Amir Mitchell.
The team first set up a screen to test the effects of 226 antibiotics and 1,054 non-antibiotics on the growth of E. coli. The non-antibiotics were defined as drugs not traditionally prescribed for bacterial infections and included approved and investigational drugs. This drug screen revealed that 104 non-antibiotics, as well as 142 of the antibiotics, significantly inhibited E. coli growth at 10 μM.
As most of the non-antibiotics were not chemically similar to antibiotics, the authors investigated their mechanisms of action in a large genetic screen. A total of 178 drugs were applied to a pooled collection of 6,700 barcoded, knockout E. coli strains covering 3,500 nonessential genes to identify gene knockouts that increased bacterial sensitivity or resistance to the drugs.
The genetic screen verified the mode of action of 90 well-characterized antibiotics. Based on this validation, a subset of informative knockout strains was chosen to perform hierarchical clustering and build a drug similarity network, which successfully grouped the antibiotics into modules reflecting the cellular pathways they impact.
By adding 77 of the non-antibiotics to this drug relatedness network, the authors found that most non-antibiotics did not cluster with the antibiotic modules, suggesting orthogonal modes of action. The non-antibiotics clustered into their own set of network modules with specific biological functions, such as metabolism, lipopolysaccharide biosynthesis and DNA repair. These clusters imply shared toxicity mechanisms and indicate potential antibacterial targets.
When knockouts for genes encoding membrane transport systems that promote drug efflux were included in the analysis, they increased the inhibitory activity of both non-antibiotics and antibiotics. This shared sensitivity indicated that non-antibiotics might potentially select for multi-drug-resistant bacteria and undermine antibiotic action.
To further probe how resistance to non-antibiotics occurs, the authors performed evolutionary selection experiments by culturing bacteria for over 200 generations with three non-antibiotics representing different network modules and sequencing the evolved bacterial clones.
The three drugs each induced independent strategies for adaptive resistance. Streptozotocin, an alkylating agent used to treat pancreatic cancer, selected for mutations in the gene encoding the glucose-6-phosphate transporter and led to fully resistant clones. Triclabendazole, used for parasite infections, led to resistant clones with an in-frame mutation in the essential gene encoding the IF2 translation initiation factor. These clones were also resistant to two other non-antibiotic drugs that bound IF2 and were neighbours in the drug relatedness network.
The third drug, the selective serotonin reuptake inhibitor sertraline, led to mutations in efflux pump regulators, which increased resistance two-fold. These evolved strains were cross-resistant to several antibiotics so had features of multi-drug resistance.
The authors note that further work is required to investigate the impact of the non-antibiotics on the human microbiome.
In future, the authors plan to increase the scale of their genetic screening capabilities and train artificial intelligence models to identify antibacterial compounds with non-canonical mechanisms of action. “We believe that such a development can resolve a key barrier in antibiotic development — the time and resources wasted on studying hit compounds from large library screens that end up working very similarly to existing antibiotics,” says Mitchell.